In the latest episode of Deployed, we talked with Maze co-founders Jo Widawski (CEO) and Thomas Mary (principal engineer) about their journey over the last year+ to make AI a core differentiator for Maze.
If you don't know it yet, Maze is a tool for user research that helps product development teams build the right product faster. A key goal for Maze is to democratize research: They help not just professional user researchers, but also designers, engineers, product managers, and product marketers who want to learn from their customers.
So you might imagine ways LLMs have already been helpful throughout their product. They’ve built a series of AI features that make the existing product experience better (rather than a separate AI experience like a chatbot). Today, AI helps Maze customers design better surveys and research studies, summarize and surface insights from survey results, and even to automatically ask follow-up questions based on a customer’s answer to a survey question.
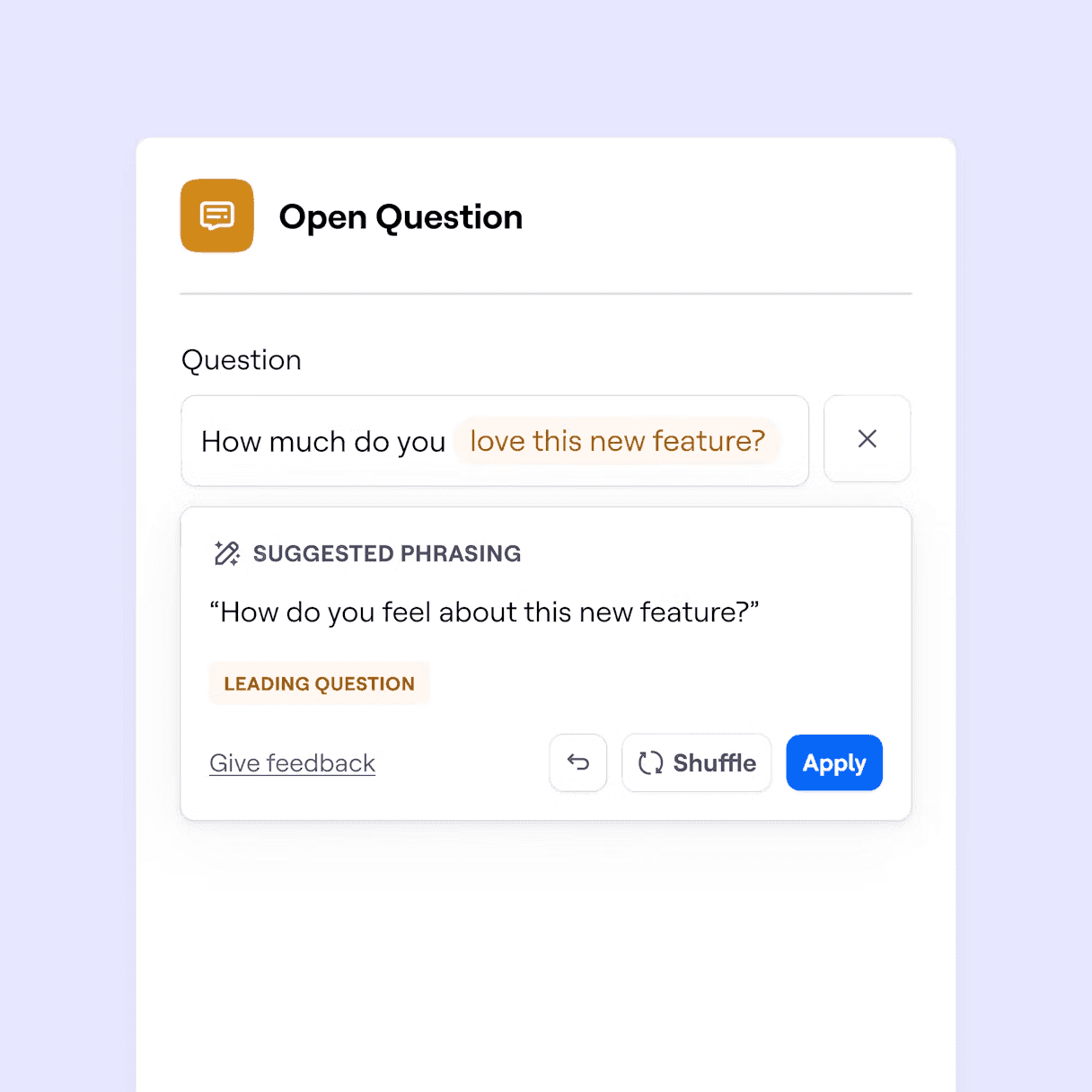
The conversation with Jo and Thomas is particularly interesting because of how deeply they think about product design, and about what it means to apply AI to solve the right problems for customers. To them, AI isn’t the point: It’s just another building material they can use to delight their customers. They’ve been intentional about using it to solve customer problems in ways that weren’t possible before.
Thomas and Jo have lots of lessons learned, and a strong vision for the role AI plays in their future. In this episode they talk about:
How they’ve approached product design with generative AI in mind, and how they’ve decided where to incorporate AI into the product
The impact their AI investments have already made on Maze as a business, and their vision for the future
Some of the challenges building in the last year — including a feature that didn’t work out, customers disabling AI features due to compliance concerns, and lessons learns with prototyping and monitoring AI features
How they’ve begun to build expertise across their engineering and product organization when it comes to creating AI product experiences
Below the video, we’ve pulled out some of our favorite clips below and share a few of our thoughts on each. If you want to jump right in, here are the show notes and the full episode. Read on for more!
4:18 - The story of Maze: Democratizing user research & helping companies build the right product faster
6:52 - The vision for AI at Maze: Helping more people do good research, and helping teams get to the right answer faster
10:35 - Practical problems that Maze solves for customers: Creating studies or surveys, recruiting people, and turning responses into insights.
15:18 - Spotting good opportunities for generative AI: How Maze has used AI to create both “radical shifts” in what’s possible, and also quick wins that create delight
18:57 - Helping researchers craft “The Perfect Question” with the help of AI
21:04 - Helping customers learn to trust AI products
30:58 - How they think about the future, and what better models will mean
32:50 - Their process to prototype and bring new AI features to life
35:46 - On selling AI features to customers, plus some objections (including why some customers turn off AI features)
38:33 - The impact of AI for Maze so far (product differentiation is at the top of the list)
40:48 - How Maze is approaching staffing for AI projects and “building the DNA” across teams
45:11 - On solving hard problems like testing and monitoring, and building a playbook for how to work on AI features
47:23 - One piece of parting advice: “Focus on solving customer problems. AI is a building piece just like everything else to deliver value.”
Incorporating AI into an existing product
When many teams start thinking about how to take advantage of LLMs, their minds jump to building a chatbot. Sometimes it’s the right answer, and sometimes it’s not! Often some of the most compelling AI product experiences are fully native within an existing product.
That’s the case with Maze’s use of generative AI (at least so far). They talk about how they identified customer problems and areas of the product where AI could make a difference:
Radical Shifts: Pains points in the customer journey where new technology could transform what’s possible
Quick Wins: Areas where Maze could easily deliver new moments of delight for customers by adopting LLMs
In Maze’s case this looks like:
A Radical Shift: Follow-up questions for customers that seem like they were part of they original survey — a superpower for researchers who have never had a way to ask follow-up questions at scale before
A Quick Win: Nudges to help rephrase survey questions to avoid bias or “leading” questions — something a human could always have done, but it’s just faster now
Watch the clip.
The biggest impact from AI so far: Differentiation
Once companies do make investments in AI, questions quickly come up about how to measure the impact — especially if you don’t sell AI features as a separate SKU. We’ve heard this discussion a lot: Is the goal retention? New sales? Increasing customer satisfaction? Simply staying competitive in a space where others are adding AI features too?
For Jo and the team at Maze, the clear leader has been sales impact. Jo talks about what it looks like to sell software In a space with a lot of incumbents: “You don’t buy the bake-off, you buy the curve.” In other words, it’s not so much about the exact features the company offers today as it is about where they’re going, and whether that product vision aligns with the buyer’s needs. Maze’s quick work to add meaningful generative AI features has helped set them apart.
How Maze thinks about helping every engineering team build with AI
“It’s not something that you outsource to a pod, it’s something that you embed in the way that teams need to be thinking. They need to incorporate that this is a technology that needs to be available to build stuff.”
So many of the leaders we talk to are trying to figure out the right way to staff their product development organization for building with generative AI. Do you build a dedicated AI team and centralize learnings and new skills in one place? Do you let each team figure it out on their own, at different rates?
Jo and Thomas talk about how they started with a dedicated “AI pod”, and then decided to split up the group and have each team be responsible for thinking about ways to use generative AI. But by splitting up the original team, they’ve also spread learnings out across the org.
And a bonus: On using Freeplay
We’re fortunate to count Maze as a customer, and Thomas gave us a quick shoutout. He talks about where Freeplay has been most helpful, including testing changes in advance of rolling them out, and helping monitor production.
Thanks so much for reading! If you’ve made it this far, you should think about subscribing for future episodes. You can subscribe on Spotify, Apple Podcasts, and YouTube.
Many thanks to both Jo and Thomas for sharing their experience with all of us. 🙌 If you’re building software products, you should definitely check out Maze to help your team gather better customer insights.
Categories
Podcast
Authors

Ian Cairns